“Our societies needed to be prepared for synthesized images becoming ever more difficult to distinguish from real ones”
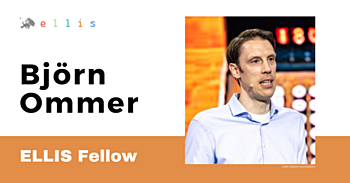
The emergence of tools like ChatGPT, DALL-E, and Stable Diffusion has brought the rapidly evolving field of generative AI into the public eye. Generative AI stands for algorithms that - starting from a certain input - create new content such as images, text or audio in a matter of seconds. The technology holds immense potential for a wide range of applications across society and industries, and is significantly influencing the way we approach content creation.
A pioneer in this field is Computer Scientist Björn Ommer who recently joined the ELLIS network as a Fellow. He works as a Full Professor at the Ludwig Maximilian University in Munich where he heads the ‘Computer Vision & Learning’ research group. Together with his team he developed ‘Stable Diffusion’, a ground-breaking open source text-to-image generator which has fundamentally expanded the possibilities of creative image generation.
In this interview, he explains why he chose to release ‘Stable Diffusion’ as an open source model, what he hopes for the future of modern AI research in Europe, and what opportunities and risks he associates with the rapid development of generative AI.
With ‘Stable Diffusion’ you and your team developed one of the most successful text-to-image generators which has been used by millions of people around the globe. You released it as an open source model free of charge. Why did you choose this approach?
When we were developing Stable Diffusion, the potential of this generative AI soon became evident. Not merely as a text-to-image generator, but for numerous other applications. Our goal was to democratize generative AI by rendering the trained model feasible on consumer hardware instead of requiring huge clusters. This promised our approach to become a widely-applicable enabling technology for future research and industrial applications. Open source was then the best path to fully unfold this enabling potential.
Secondly, it seemed obvious that AI-based image generation would improve rapidly. Thus, our societies needed to be prepared for synthesized images becoming ever more difficult to distinguish from real ones. Giving ordinary users the opportunity to create images with just a few words communicates this much better than merely telling people.
Lastly, big training data is the key ingredient to large-scale machine learning. However, there was comparably little discussion about the origin and implications of its use in closed-up models and big tech. Providing society with an inside view of the training process helped to have a public discussion about data sovereignty.
ELLIS published a statement on the need for computational resources in Europe, urging to establish an intergovernmental, multi-centric AI research organization. You are working at the forefront of AI research. How do you perceive the current situation in Europe? What is good? What should be improved?
Compute resources are a critical commodity for advancing AI research and deploying it to industry. Becoming overly dependent on companies outside Europe is posing increasing structural risks, especially considering the huge importance for the entire technological ecosystem. Therefore, pan-European as well as national initiatives to establish a sovereign infrastructure will be key, as has been convincingly proposed in the ELLIS statement. It is good that this topic is now broadly discussed in Europe.
When a new and powerful technology is rapidly becoming pervasive with a broad impact on society, the transition should be integrative and to the benefit of the people. This democratic striving is one of the core values in Europe. However, there are never perfect guarantees in all details, and aiming for such can quickly lead to excessive bureaucracy and over-regulation. The resulting slowing down of decisions is particularly critical in the highly-dynamic, internationally competitive environment of generative AI.
You did your postdoc in the United States and then continued your research career at German universities. What makes Europe attractive for you as a scientist?
Holding a chair at a German university comes with valuable scientific freedom and independence. With AI research now having immediate implications on society, I consider it important that there are independent voices from academia that can autonomously explore and discuss critical aspects.
Increasingly powerful AI systems can lead to great benefits for society, but they are also associated with fears and challenges - from algorithmic discrimination, lack of transparency and excessive carbon footprint to existential risks. Which perspective do you have on the benefits and risks emerging from the rapid advances in AI? Do you miss any particular aspect in the public debate?
At times, I am missing a nuanced discussion that strikes a balance between both extremes. Moreover, the assumptions underlying these positions should be discussed more openly rather than simply jumping to conclusions. Evidently, polarizing views draw a lot of attention. But only when we start with our common values, rather than with what divides us, we can come to a compromise. Overall, I think that generative AI will render the PC more powerful and easier to use. When we keep misuse of this technology in check, users will be able to address much more complex problems and develop tailored solutions. The interaction between users and computers will become more natural and the AI will make information much easier accessible by presenting it according to the knowledge background of the user.
What are your current research projects at the Maximilian University in Munich?
I find it fascinating to continue our vision from Stable Diffusion and democratize AI by rendering smaller models more effective. Intelligence results when we have to learn and cope with finite resources. My group is adding more precise and yet convenient control to visual synthesis, and we extend this to video generation and 3D. Moreover, I believe the future to not just belong to better performing AI, but to one that can collaborate with human users naturally. For that and as a basis for broad acceptance of AI it is important for it to be interpretable and transparent. In addition, my group has for long been exploring deep metric and representation learning, which are key to establish semantic relationships between images.
Taking a closer look at your career path: What motivated you to go into AI research? What is keeping you there?
Man had been on the Moon, on the highest mountains and on the bottom of the ocean. All white spots on the maps seemed to have been charted. So as a kid I was wondering what was left and worth exploring. I then found our human intelligence to be one of the greatest unsolved mysteries. I was fascinated to not just speculate about this open frontier, but rather to understand tiny aspects thereof by artificially reproducing some of our cognitive abilities. However, despite all progress in our field, I feel that this mechanistic approach may uncover important principles. Nevertheless, artificial intelligence is otherwise as close to our brain as a noisy, kerosene-swallowing jet airliner is to a common swift.
About Björn Ommer
Björn Ommer is an ELLIS Fellow, a Unit Faculty at the ELLIS Unit in Munich, and a Full Professor at the Ludwig Maximilian University (LMU) of Munich where he heads the ‘Computer Vision & Learning’ research group. Previously, he was a Full Professor at the Department of Mathematics and Computer Science at the University of Heidelberg. He received his diploma in Computer Science from the University of Bonn, his PhD from ETH Zurich, and worked as a Postdoc at UC Berkeley. His research interests cover all aspects of semantic image and video understanding based on (deep) machine learning. Together with his research team he developed the open source ‘Stable Diffusion’ text-to-image generator which has fundamentally expanded the possibilities of creative image generation.
More information
The science behind ‘Stable Diffusion’:
ommer-lab.com/research/latent-diffusion-models
The ‘Computer Vision & Learning’ group at LMU:
ommer-lab.com/people
More about ELLIS Fellows and how to join ELLIS as a Fellow:
https://ellis.eu/fellows
Website of the ELLIS Unit Munich:
https://www.ellismunich.ai
ELLIS statement on the need for computational resources in Europe:
ellis.eu/news/ai-foundation-models-a-roadmap-for-europe
The view of the ELLIS Board on the global conversation about the societal risks of AI
ellis.eu/news/our-view-on-the-global-conversation-about-the-societal-risks-of-ai
Follow ELLIS
Follow ELLIS on Twitter, LinkedIn, Mastodon and Facebook.
Subscribe to the ELLIS newsletter here.
Several AI networks in Europe build on ELLIS, connect researchers across different fields, and offer training and mobility programs for scientists. Get an overview here or follow ELISE, ELSA, ELIZA and ELIAS to learn more about the opportunities.
Contact
Contact ELLIS at pr@ellis.eu