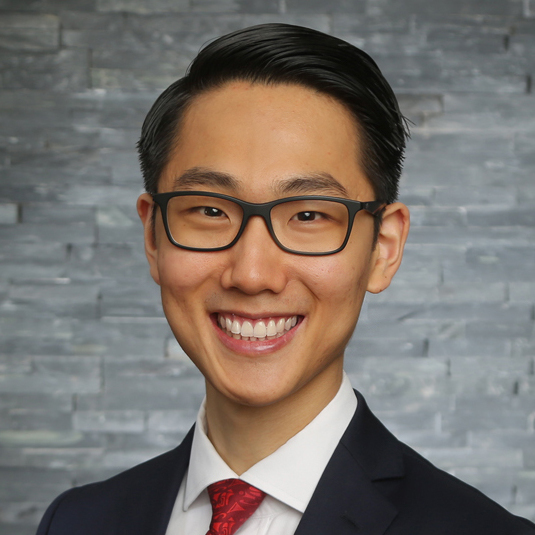
Uncertainty Quantification in Deep Learning
Jihao Andreas Lin (Ph.D. Student)
In recent years, deep learning has achieved remarkable success at advancing complex tasks, such as computer vision and natural language processing. While deep learning models and algorithms are already being used in some industry and consumer applications, a lack of interpretability and trustworthiness is obstructing employment in sensitive environments and domains like traffic or medicine. To be interpretable and trustworthy, it is imperative for a deep learning model to be capable of quantifying the uncertainty of its predictions. In particular, this uncertainty quantification should be well-calibrated such that the model is confident about its prediction if it is correct and uncertain if the prediction cannot be reasonably inferred from the observed data (epistemic uncertainty) or if the prediction is inherently noisy (aleatoric uncertainty). Therefore, an overarching research question of interest to me is: How can we create universal function approximators, such as neural networks, with well-calibrated uncertainty estimation?
Primary Host: | José Miguel Hernández-Lobato (University of Cambridge) |
Exchange Host: | Bernhard Schölkopf (ELLIS Institute Tübingen & Max Planck Institute for Intelligent Systems) |
PhD Duration: | 01 October 2022 - 30 September 2026 |
Exchange Duration: | 01 October 2025 - 30 September 2026 - Ongoing |