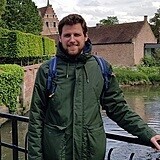
Uncertainty estimation in deep neural networks
Raphael Sayer (Ph.D. Student)
In many classification tasks in machine learning not just the predicted label but also the estimated probability of that label is of interest. While regularly achieving high prediction accuracies, deep neural network classifiers usually ouput label probabilities that do not match the true underlying probability distribution. In my PhD project I aim to overcome this pitfall by developing well calibrated deep learning architectures. These are models whose predicted probabilities reflect the true label distribution and hence these probabilites can directly be interpreted as uncertainty estimates or confidence levels. I will apply these calibrated deep neural networks in the context of image segmentation and remote sensing.
Primary Host: | Matthew Blaschko (KU Leuven) |
Exchange Host: | Philipp Hennig (University of Tübingen) |
PhD Duration: | 01 April 2021 - 01 April 2025 |
Exchange Duration: | 01 April 2023 - 30 September 2023 - Ongoing |