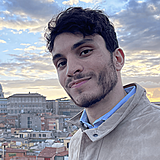
Representation learning through the lens of geometry
Marco Fumero
My research stands at the intersection of geometry and representation learning. Specifically, I am interested in two different directions: the former is focused on developing algorithms for geometrical data, i.e. data lying on non-euclidean domains such as 3d data, point clouds, and graphs, with the aim of finding general, explainable and reusable representations for different tasks. The main challenge is to obtain representations which must be invariant to different classes of deformation, incompleteness, and topological noise, typical of real data distributions, as well as the challenge to work in a low data regime and mostly unsupervised setting, due to the lack of labeled data. This direction intersects different fields such as geometry processing, computer vision, shape analysis and representation learning. For the latter direction, my aim is to incorporate geometrical priors in general machine learning models (agnostic to the data modality) in order to build more robust and interpretable models. Regularizing the space of solutions to fit specific geometrical structure enables indeed to work in a low data regime, and generalize well in an out of distribution setting. Moreover, exploiting the geometrical structure is possible to understand and interpret the representations learned by a neural network, and obtain sparse and disentangled components with respect to the core attributes of the data one is interested to capture, i.e. recover the factors of variation underlying the data distribution. This direction intersects fields such as disentangled representation learning, few shot learning, geometry processing and domain generalization.
Primary Advisor: | Emanuele RodolĂ (Sapienza University of Rome) |
Industry Advisor: | Francesco Locatello (IST Austria) |
Project Duration: | 01 November 2019 - 01 November 2023 |