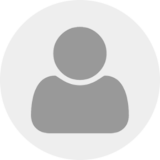
Noise-Aware Differentially Private Synthetic Data
Ossi Räisä (Ph.D. Student)
Differential privacy (DP) is currently considered the gold-standard for privacy preserving data analysis. A particularly promising application of DP is synthetic data generation, where a synthetic facsimile of a real dataset is released with a DP guarantee. The synthetic dataset can then be used for arbitrary downstream analyses. However, while machine learning models can be trained and statistical quantities can be estimated using synthetic data, there is very little work in estimating the uncertainty of either the learned model's predictions or the statistical estimates. The standard non-DP methods that treat the synthetic data as real da not provide accurate results, as they da not take the noise in the synthetic data generating process into account. This PhD project proposes to develop solutions to this problem, both in the form of techniques to compute uncertainty estimates from synthetic data accurately, and methods to generate synthetic data that allow the use of such methods. These methods allow widespread release and analysis of privatised synthetic datasets in privacy-sensitive areas, such as medical or insurance data, where publicly releasing any original data is not possible, and any analysis must be subjected to a potentially lengthy approval process. By working on both of these areas, 1 hope to improve how we create personalized content and expand the use of generative models in practical tasks.
Primary Host: | Antti Honkela (University of Helsinki & Finnish Centre for AI) |
Exchange Host: | Mihaela van der Schaar (University of Cambridge, The Alan Turing Institute & University of California) |
PhD Duration: | 01 June 2021 - 31 December 2025 |
Exchange Duration: | 01 September 2024 - 01 March 2025 - Ongoing |