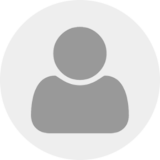
Multitask Visual Representation Learning
Duy-Kien Nguyen (Ph.D. Student)
In order to build a comprehensive vision system at the human level, it is necessary for the system to be able to deal with multiple problems at different levels. Previously, researchers have focused on individual tasks separately with a specific network architecture design and many hand-crafted modules, which makes it more difficult to generalize across tasks. In this research, I aim to create a unified system which learns visual representations in an end-to-end manner from diverse datasets of different vision problems. Specifically, we start by designing a robust neural architecture for end-to-end representation learning. The designed architecture can easily adapt to a wide range of vision problems (i.e., 2D and 3D object detection, segmentation). It is our plan to explore the potential of multitask learning which allows the network to perceive visual information in an unified manner. The final step of our project is to tackle self-supervised learning in detecting objects. By utilizing a huge amount of unlabeled data generated in the real world, we expect the system to reach human-level performance.
Primary Advisor: | Cees Snoek (University of Amsterdam) |
Industry Advisor: | Olaf Booij (TOMTOM) |
PhD Duration: | 19 October 2020 - 19 October 2024 |