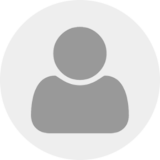
Multilinear re-parametrizations of Deep Neural Networks
Thomas Christie (Ph.D. Student)
Deep neural networks are heavily (over-) parametrized families of probability distributions. They are naturally endowed with a metric given by the curvature of the training loss, and thus form Riemannian manifolds. In principle this mechanism allows the definition of a probability measure on the weights, and the predictive output, of the neural network. However, the metric is not invariant under changes to the parametrization of the weight space. A natural and quite fundamental question thus arises: What is the right parametrization — and thus the right posterior, to assign to a deep neural network? In his PhD project, Thomas Christie will initially focus on the parametrization of linear neural networks as a starting point. In these models, depth is a free and entirely virtual concept, which allows close analytic and efficient practical study of the effect of parametrization. Based on insights from this opening project, we may focus on the effect of standard non-linear link functions (like ReLU) or on other parts of the parametrization. The ultimate goal is to identify practical and useful parametrizations of deep neural networks that faithfully represent epistemic uncertainty. We will work towards this goal in collaboration with other, parallel projects across ELLIS, in Tübingen, København, Cambridge, and elsewhere.
Primary Host: | Philipp Hennig (University of Tübingen) |
Exchange Host: | Carl Henrik Ek (University of Cambridge) |
PhD Duration: | 01 October 2024 - 30 September 2027 |
Exchange Duration: | 01 July 2025 - 30 September 2025 01 July 2026 - 30 September 2026 |