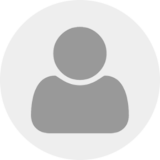
Learning with Geometry on real world data
Marco Pegoraro (Ph.D. Student)
This PhD project delves into harnessing the intrinsic geometric properties of data to amplify learning tasks, with a primary focus on biological data. Traditional Euclidean models often prove inadequate in capturing the intricate structures inherent in real-world data. Consequently, we delve into nuanced spaces that better encapsulate these properties, fostering more efficient learning processes. From a Geometry processing perspective, spectral representation emerges as a pivotal tool for shaping our exploration. We investigate its utility in both shape modelling and graph isomorphism. In shape modelling, we pioneer novel methods by combining eigenvalues computed on different levels, thereby amalgamating features from diverse 3D shapes. Concurrently, in graph isomorphism, we exploit the potential of spectral representation to elucidate the correspondence between graphs and their various sub-isomorphic levels, leading to significant enhancements in learning tasks. On the other hand, we leverage classical tools from geometry processing and probability theory to extend quantile computation to manifolds. This exploration extends to practical applications across real-world datasets, ranging from climate measurements to dihedral angles in proteins. The apex of this research manifests in the application of geometric deep learning principles to biochemistry, with a dedicated focus on unravelling the intricate structures of small molecules and proteins. By seamlessly integrating geometric insights into the learning process, this project achieves substantial progress in deciphering the complexities of biological data and elevating predictive accuracy. A prime example of this is our investigation into geometric deep learning applied to antibodies and antigens, where we leverage intrinsic graph structures and outer surface characteristics to achieve superior performance. In summary, this project underscores the transformative potential of integrating geometric information into machine learning frameworks, particularly within the realm of biological data analysis. A blend of innovative methodologies and rigorous empirical validations delivers significant contributions to theoretical understanding and practical applications in computational biology and bioinformatics.
Primary Host: | Emanuele RodolĂ (Sapienza University of Rome) |
Exchange Host: | Alex Bronstein (Technion) |
PhD Duration: | 01 November 2021 - 30 September 2025 |
Exchange Duration: | 01 March 2023 - 01 August 2023 - Ongoing |