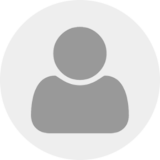
Learning-based 3D Approach for Egocentric Vision
Weirong Chen (Ph.D. Student)
Egocentric vision, which involves understanding and interpreting visual data from a first-person perspective, is crucial for various applications, including augmented reality, robotics, and assistive technologies. This project seeks to advance the current state of visual foundation models while addressing challenges associated with the efficiency and scalability of 3D processing, robustness in dynamic scenes, and 3D world synthesis. A critical research problem in this context is recovering the camera pose and dynamic 3D environment from egocentric inputs. To augment the precision and efficiency of current Structure from Motion (SfM) methods, we plan to incorporate learning-based approaches into the visual-inertial Simultaneous Localization and Mapping (SLAM) framework for egocentric videos, for example, DM-VIO. This integration is expected to provide robust camera tracking and 3D recovery even under rapid camera movements and complex object motions. Another area of focus will be the synthesis of dynamic 3D scenes, addressing challenges such as the lack of 3D data, the scaling problem from 2D to 3D, and the transition from object-level to world-level synthesis. We plan to use EPIC-KITCHENS and EPIC Fields datasets as the primary resources. Containing various daily activities captured from a first-person viewpoint, they will be instrumental for training and validating the developed models.
Primary Host: | Daniel Cremers (Technical University of Munich) |
Exchange Host: | Andrea Vedaldi (University of Oxford) |
PhD Duration: | 01 October 2023 - 30 September 2027 |
Exchange Duration: | 01 February 2025 - 31 July 2025 - Ongoing |