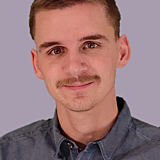
Interpretable Machine Learning
Jonas Klesen (Ph.D. Student)
Issues around the interpretability of Machine Learning systems are of growing concern. Unfortunately, the research community up until now has failed to converge on established methods for arriving at powerful yet interpretable models. Even worse, and as opposed to similar desiderata of Machine Learning systems, such as robustness and privacy, there is not even consensus on how to properly define let alone measure the interpretability of a Machine Learning system. My thesis strives to not only make progress on methods for arriving at interpretable machine learning models, but also on the underpinnings of the research area, including its raison d’etre, its metrics and its definitions.
Primary Host: | Isabel Valera (Saarland University & Max Planck Institute for Intelligent Systems) |
Exchange Host: | Novi Quadrianto (University of Sussex) |
PhD Duration: | 01 September 2021 - 01 September 2024 |
Exchange Duration: | - Ongoing - Ongoing |