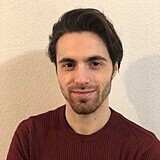
Human-centric Explainable AI
Julien Colin (Ph.D. Student)
The goal of Explainable AI (XAI) is to develop methods that explain to humans how AI models behave so humans (including non-experts) can understand the basis for the algorithm’s decisions. Within the computer vision literature, the most widely used explainable AI methods are attribution methods. Recent works on human evaluation of attribution methods in computer vision have highlighted that, (1) they seem to be good enough to explain ‘where’ the model is looking. However, there is an opportunity to expand the focus of the XAI field to the development of complementary explainability methods that tell us ‘what’ the model is seeing; and (2) we need new, human-centric benchmarks to score explainability methods as those currently used in the field are too disconnected from human cognition and understanding. In this PhD, we propose to address the two previously described areas of opportunity by proposing the use of generative models to develop novel explainability methods that complement the limitation of current attribution methods, and by taking inspiration from neuroscience to develop benchmarks that give us better insights into the practical utility of explainability methods. We plan to evaluate the proposed approaches both on benchmark datasets and real-life scenarios.
Primary Host: | Nuria Oliver (ELLIS Alicante Unit Foundation | Institute of Humanity-centric AI) |
Exchange Host: | Thomas Serre (Artificial & Natural Intelligence Toulouse Institute & Brown University) |
PhD Duration: | 01 November 2022 - 30 June 2026 |
Exchange Duration: | 01 June 2024 - 31 December 2024 - Ongoing |