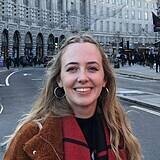
Geometric and statistical software engineering tools in array-centric programming
Joanna Sliwa (Ph.D. Student)
In the course of training neural networks, the evaluation of the training process is often solely based on the determined loss. Prior research has attempted to incorporate software engineering tools to enable an assessment beyond just the gradient, perform it efficiently, and offer a more comprehensible evaluation of the training process. In our current project, we aim to leverage Laplace approximations for software engineering in deep learning to study what happens during training in neural networks. Recently, there's been a renewed interest in employing Laplace approximations to convert any deep learning network into a Gaussian Process (GP). The application of this technique could be advantageous in the scenario of multi-task continual learning, where it could mitigate the problem of catastrophic forgetting.
Primary Host: | Philipp Hennig (University of Tübingen) |
Exchange Host: | José Miguel Hernández-Lobato (University of Cambridge) |
PhD Duration: | 15 June 2023 - 14 June 2026 |
Exchange Duration: | 01 June 2024 - 01 December 2024 - Ongoing |