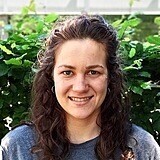
Fair decision making: From learning to predict to learning to decide
Miriam Rateike (Ph.D. Student)
With algorithmic decision making processes being increasingly being deployed in society, there are growing concerns about potential unfairness of these algorithmic systems towards people from certain demographic groups (e.g., gender). To address these concerns, the emerging field of ethical machine learning has proposed quantifiable notions of fairness as well as mechanisms for ensuring fair and unbiased algorithmic decision making. However, the negative consequences of feedback loops between the observed data and decision making process has largely been ignored to date. In this project, we aim to contribute to automatic fair decision making by explicitly accounting for the feedback loop induced by the decision policy when designing machine learning models. To this end, we first focus on the selective labels scenario and how different approaches to fairness can be adapted to correct for the selective labeling bias. Second, we will develop (deep) generative models to capture the joint distribution of the observed non-sensitive attributes, the sensitive attribute, the label and the decision making policy. This will allow us to cast the fair decision problems into policy learning tasks, allowing to leverage and extend (to account for fairness constrains) the literature in counterfactual learning and contextual bandits to solve that problem. Third, we will use and generalize the developed generative models to study the impact of different fairness policies in the joint distribution. As a result, we will be able to better estimate and understand the delayed impact of fair machine learning.
Primary Host: | Isabel Valera (Saarland University & Max Planck Institute for Intelligent Systems) |
Exchange Host: | Max Welling (University of Amsterdam) |
PhD Duration: | 15 July 2020 - 14 July 2023 |
Exchange Duration: | 01 June 2022 - 31 December 2022 - Ongoing |