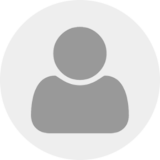
Explainable De-biasing in Learning from Interactions
Maria Heuss (Ph.D. Student)
Explainability and Fairness are two topics of great importance when working with machine learning models that might not be intrinsically transparent. In this project we are looking a these topics in the context of ranking systems. The nature of user behavior, when interacting with ranking systems, is inherently biased with respect to how much attention is given to each item in the ranked list, and hence poses a challenge specific to this ML application, with respect to fairness. In this project we aim to develop fair ranking systems and investigate certain assumptions that are made when defining such fair solutions and improve on previous methods in cases where those assumptions might not hold. Furthermore, we explore how to make these ranking systems more explainable. Even a perfectly fair ranking systems might not be trusted by the user if it the decisions made by the system can not be understood by the user. Explainability has made big advances in other fields, nevertheless explaining ranking systems remains a rather under-explored field. Certain aspects about ranking models make the task of explaining these models especially difficult. So is the output of a ranking model based on the ranking scores of several items, rather than just a single prediction. In this project we explore ways to explain such listwise rankings and how to evaluate the quality of such explanations.
Primary Host: | Maarten de Rijke (ICAI & University of Amsterdam) |
Exchange Host: | Carlos Castillo (Universitat Pompeu Fabra) |
PhD Duration: | 01 November 2020 - 01 March 2025 |
Exchange Duration: | 01 October 2023 - 01 April 2024 - Ongoing |