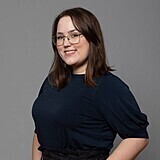
Essentials for few-shot learning on images
Jelena Bratulic (Ph.D. Student)
Few-shot learning strives to imitate the ability of humans to learn how to recognize an object after only having seen a small number of samples of the new object. This is achieved by leveraging the rich world model that humans have learned over their life span to explain novel patterns as variations of known patterns. In contrast, most machine learning methods today require thousands of labeled data samples to learn how to recognize a new class. This severely limits their scalability to new classes and, more fundamentally, limits their applicability to many real-world scenarios in which building large-scale datasets is intractable. The core principle of few-shot learning is to build on visual feature representations obtained by pre-training neural networks on large image datasets and transfer the learned representation to recognize new object classes from a few samples in a data-driven approach. We will develop a deeper understanding by investigating the various strategies to pre-train deep networks, including self-supervised and vision-language-based training strategies, and their effects on few-shot learning. Moreover, we will develop new core algorithms for few-shot learning, including matching strategies for few-shot learning, and generative techniques to learn residual features for distinguishing new objects.
Primary Host: | Thomas Brox (University of Freiburg) |
Exchange Host: | Christian Rupprecht (University of Oxford) |
PhD Duration: | 01 September 2023 - 30 June 2027 |
Exchange Duration: | - Ongoing - Ongoing |