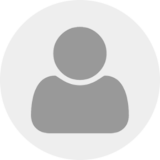
Enabling complex computer vision tasks on the edge: applications to semantic segmentation and beyond
Claudia Cuttano (Ph.D. Student)
Most of the research in Deep Learning for Computer Vision is dedicated to the development of novel, more and more complex and overparametrized architectures which increase state of the art accuracy in solving specific tasks. However, this approach lays its foundations on the strong assumption that it is always possible to increase the energetic and computational demand w.r.t. previous implementations. Yet, when it comes to the deployment of existing solution in realistic use cases, most of the available solutions cannot be used simply because they require high-end hardware such as GPUs even for inference, which may represent a major problem for battery-powered implementations such as cars, drones, satellites, robots. The inefficiency of existing solutions not only has an impact on the energetic and memory budget, but also requires a large amount of annotated data for training, which is time consuming and costly to gather. The goal of this research is to provide solutions for increasing the efficiency of deep learning models in terms of resources (data, memory, energy) needed for training and testing. At a first stage, particular attention will be given to complex computer vision tasks, such as semantic, instance and panoptic segmentation. Then, the research will extend the investigation to multi-modal settings, where images are used as data input together with other data sources, such as text. We will study how to use large pretrained models as source for the distillation of knowledge into tiny architectures, eventually considering semi or weekly supervision as an alternative to fully supervised training, to increase dataefficiency of models and minimize the need of large-annotated datasets.
Primary Host: | Giuseppe Averta (Politecnico di Torino) |
Exchange Host: | Stefan Roth (Technical University of Darmstadt) |
PhD Duration: | 01 February 2023 - 31 January 2026 |
Exchange Duration: | 01 January 2025 - 30 June 2025 - Ongoing |