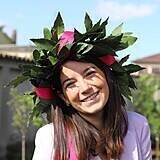
Cross-domain activity classification from multiple information channels
Chiara Plizzari (Ph.D. Student)
Recognizing human actions from videos is one of the most critical challenges in computer vision since its infancy. An open challenge is that video analysis systems heavily rely on the environment in which the activities are recorded, which inhibits their ability to recognize actions when they are recorded in unfamiliar surroundings or in different lighting conditions. This problem, known in the literature as domain shift, has long been studied in the image classification domain and has recently started to attract attention also in the activity classification community. In this thesis, we investigate cross‐domain video analysis for activity classification. Most of the researchers in the field addressed this issue by reducing the problem to an unsupervised domain adaptation (UDA) setting, where an unlabeled set of samples from the target is available during training. We aim to also address the so-called Domain Generalization (DG) setting, consisting in learning a representation able to generalize to any unseen domain, when target data are not accessible at training time. Taking inspiration from recent works on self‐supervised audio‐video processing, we investigate how to solve auxiliary tasks across various information channels from videos in a way that makes the solution of such tasks consistent across information channels and gains robustness from it. We explored the use of unsupervised domain adaptation techniques in both third person and first person scenarios, where the camera is mounted on the observer and it moves around with her. The recent problem of Unsupervised Domain Adaptation has been also introduced in Cross-modal Video Retrieval tasks, where a visual domain gap exists between the captioned video sequences and the gallery of videos we wish to retrieve from. In our analysis, we consider both standard RGB videos, where the motion and appearance channels can be seen as different information streams, as well as videos acquired with multiple modalities, from audio‐visual signals to skeleton sequences and text. Additionally, we also explore the possibility to use event data in combination to the standard RGB modality. Indeed, event cameras are novel bio-inspired sensors, which asynchronously capture pixel-level intensity changes in the form of “events". Their high pixel bandwidth and high dynamic range, together with their low latency and low power consumption, make them perfect to tackle well-known issues which arise from the use of wearable devices, such as fast camera motion and background clutter, which are typical of egocentric action recognition tasks.
Primary Host: | Barbara Caputo (Politecnico di Torino & Italian Institute of Technology) |
Exchange Host: | Dima Damen (University of Bristol) |
PhD Duration: | 01 September 2020 - 31 March 2024 |
Exchange Duration: | 29 August 2022 - 27 November 2022 - Ongoing |