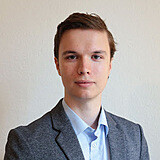
Bayesian deep learning for medical time series
Fedor Sergeev (Ph.D. Student)
Deep learning (DL) has seen tremendous growth in the last 20 years and enabled rapid advances in computer vision, natural language processing, and robotics. However, the direct application of DL methods to healthcare is challenging due to the nature of medical data. Creating specialized data sets for DL in medical applications is complicated due to cost, ethical regulations, and non-uniform clinical practices. One area where big healthcare data is available is the prediction tasks for Intensive Care Units (ICU). Such data is high-dimensional, noisy, and multimodal (contains time series, text, tabular data, etc.). In addition to simply dealing with such complex data, medical applications require the models to provide uncertainty estimations and be robust and interpretable. Bayesian deep learning (BDL) is an extension of DL that combines the flexibility of deep neural networks with the possibility of uncertainty estimation from Bayesian statistical models. Therefore BDL models are particularly promising for applications to medical data as they can be used on complex time-resolved data and provide uncertainty at the same time. Additionally, BDL offers a principled framework for incorporating prior medical knowledge into the DL models, potentially making them more data-efficient, accurate, or reliable. We aim to investigate along the whole Bayesian pipeline (priors, model, inference) and develop state-of-theart BDL methods for tasks such as early-event prediction, imputation, and concept discovery on medical time series. We will apply the developed methods on the latest ICU datasets (MIMIC-III, HiRID, IICU) and compare them to other SOTA BDL methods (Deep Ensembles, MultiSWAG, LANAM, etc.). Our work will contribute to the fields of medical Artificial Intelligence, Bayesian deep learning, and representation learning.
Primary Host: | Gunnar Rätsch (ETH Zürich) |
Exchange Host: | Vincent Fortuin (Helmholtz AI) |
PhD Duration: | 01 July 2023 - 01 December 2027 |
Exchange Duration: | - Ongoing - Ongoing |