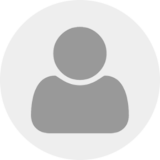
A causal approach to privacy, fairness and distributional robustness in machine learning
Yaxi Hu (Ph.D. Student)
While machine learning becomes an integral component of our daily life, people often overlook the profound consequences these models pose, especially concerning the privacy of the training data and fairness towards its users. Central to solving these problems is the challenge of ensuring generalization under distribution shifts. Specific privacy threats, such as membership inference attack, jeopardize the privacy of training samples primarily due to model overfitting and inability to accurately generalize from the sampling distribution to the population distribution. Concurrently, fairness issue in machine learning is largely due to the models’ inconsistent generalization across different population groups, leading to potential bias and discrimination. This PhD project seeks to address these challenges simultaneously with causality. Our objectives are two folds: 1) to understand information leakage from the training data of machine learning models from a causal perspective, 2) to design methodologies that exploit the causal structure of the data to achieve privacy, fairness and distributional robustness simultaneously.
Primary Host: | Bernhard Schölkopf (ELLIS Institute Tübingen & Max Planck Institute for Intelligent Systems) |
Exchange Host: | () |
PhD Duration: | 10 July 2023 - 09 July 2026 |
Exchange Duration: | 20 June 2025 - 20 December 2025 - Ongoing |