Successful Finale: Machine Learning for Molecules Workshop
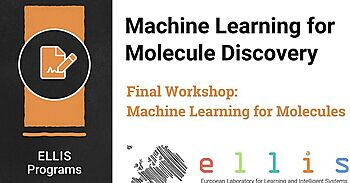
Advances in machine learning have shifted the fields of molecular and materials science, enabling novel approaches to drug discovery, materials design, and computational chemistry. Workshops like the ML for Molecules and Materials in the Era of LLMs provide a crucial platform for researchers to share insights, collaborate, and explore how these technologies are shaping the future of AI research.
The ML for molecules and materials in the era of LLMs workshop, part of the ELLIS Machine Learning for Molecule Discovery program, was a prominent event held on December 6, 2024, at FHI Berlin and virtually. It brought together experts to discuss innovations in molecular machine learning and attracted wide attention with over 700 registrations globally, 50 on-site attendees, and 350 unique online participants. The agenda included diverse topics and the following speakers.
Invited Talks:
- Tim Duignan (University of Cambridge): Neural network potentials solve the connecting scales problem.
- John Chodera (Memorial Sloan Kettering Cancer Center): Teaching free energy calculations to learn.
- Cecilia Clementi (Rice University): Modeling protein dynamics with machine learning and molecular simulation.
- Daniel Probst (University of Bern): Less is More.
- Igor Tetko (Helmholtz Zentrum München): Expectations, achievements, and lessons of AIDD and AIChemist Marie Skłodowska-Curie Innovative Training Network - European Industrial Doctorate projects.
- Jutta Rogal (University of York): Mapping phase diagrams with deep generative models.
- Jian Tang (Mila): Geometric Deep Learning for Protein Design.
Best Paper Awards sponsored by AstraZeneca:
- DEQuify your force field by Andreas Burger et al.: Highlighted the efficiency in simulations using deep equilibrium models.
Bio-xLSTM by Niklas Schmidinger et al.: Discussed generative modeling and sequence representation for biological and chemical systems.
Contributed Talks and Posters:
- Topics ranged from retrosynthesis modeling to nanoparticle design, equivariant diffusion models for reaction states, and ligand docking.
- Posters explored cutting-edge methods in activity prediction, inverse catalyst design, and high-throughput screening.
Networking and Collaboration:
Two poster sessions facilitated discussions among attendees on topics such as graph neural networks, retrosynthetic planning, and molecular docking.
This workshop underscored the critical role of machine learning in accelerating advancements in drug discovery, materials science, and computational chemistry. For more details, including a comprehensive agenda and speaker abstracts, visit the official workshop page.