Shaping the Future of 3D: Insights from the ELLIS Workshop on 3D Vision and Graphics 2025
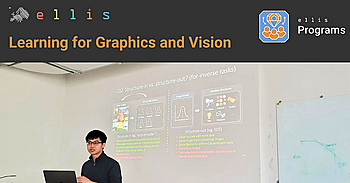
The members of the ELLIS Program ‘Learning for Graphics and Vision’ hosted a workshop on March 20-21 on 3D Vision and Graphics at the Tübingen AI Center in Tübingen, Germany. This event brought together leading researchers of the field to discuss the rapidly evolving capabilities of image and video generative models and their implications for 3D understanding.
Gerard Pons-Moll, ELLIS Scholar, Co-Director of the ELLIS Program Learning for Graphics and Vision, and one of the event’s organisers, emphasised the growing relevance of video and image generative models in both research and industry. “Impressive multiview and video synthesis is achieved without explicit 3D representations,” he noted. “This trend raises important questions about the future role of explicit 3D models.”
Generative models are increasingly capable of producing realistic images and videos from minimal inputs, without relying on hand-crafted 3D representations. As in language modeling, large-scale data and compute are beginning to replace explicitly designed models. However, this shift sparked valuable discussion on the enduring relevance of explicit 3D representations. Participants highlighted their efficiency, lower data demands, built-in 3D coherence, and reduced computational footprint. In this context, explicit models were seen as a promising long-term foundation for building robust, controllable, and interpretable 3D world models.
The talks also explored how to harness the power of image and video foundation models to extract 3D information and ensure multiview consistency - key drivers of innovation in 3D vision and graphics.
Andrea Tagliasacchi (Simon Fraser University, Google) presented his work on Monte-carlo Neural Rendering, offering insights into probabilistic approaches for rendering complex scenes.
Andreas Geiger (University of Tübingen, ELLIS Fellow and member of the Machine Learning and Computer Vision program) discussed feed-forward fast Gaussian Splatting based representations.
Federico Tombari (Technical University of Munich, Google) shared advances in cubemap synthesis frameworks for efficient 3D scene generation.
Vincent Lepetit (Ecole des Ponts ParisTech) introduced a novel method that uses decision trees, inspired by AlphaGo, to prune object detection candidates, improving accuracy and efficiency.
Gerard Pons-Moll (University of Tübingen), alongside the other speakers, examined the strengths and trade-offs of different 3D representations, including NeRF-based methods, Gaussian Splatting, and hybrid models, and discussed the potential of feedforward networks to directly predict 3D scenes and layouts from images and scans.
The workshop also included talks by Hendrick Lensch (University of Tübingen) recapping his recent research on inverse PBR lighting. Victor Lempitsky (Skoltech) presented a talk that surveyed the evolution and techniques of gathering immersive VR content by weaving together historical methods with modern advancements. Additionally, Jan Eric Lenssen (Max Planck Institute for Informatics) discussed how AI models can learn to reason about and generate spatial structures, pushing the boundaries of geometric deep learning.
Gül Varol (École des Ponts ParisTech, ELLIS Scholar and member of the ELLIS Unit Paris), Elliott Wu (University of Cambridge), and Gerard Pons-Moll presented talks exploring the learning of advanced 3D models of animals, objects, and humans. Gül focused on text driven human motion, while Elliott shared his work titled "From Pixels to 3D Motion: Modeling the Physical Natural World from Images", which investigates how to infer 3D dynamics from visual data. Gerard discussed techniques for learning 3D models that can be controlled using multimodal inputs such as text, video, and single images, emphasizing the importance of interactivity and interpretability in 3D understanding.
“We discussed the fascinating tension between the increasing capabilities of purely generative models and the inherent advantages of explicit 3D representations,” Gerard explained. “While large-scale data and compute are undeniably powerful, we emphasized that explicit 3D representations offer greater efficiency, require less data, and naturally ensure 3D coherence. We also discussed the critical importance of considering the computational footprint of our models. In the long run, explicit 3D representations are the best bet to make 3D world models controllable, interactive, and robust.”
Niloy Mitra (University College London) spoke about the role of neural networks for representing surfaces and geometry processing. Andreas Taggliasacchi said, “I particularly liked Niloy's talk, as it bridges my past (geometry processing) with my current expertise (neural fields). I find the way in which he was able to compute Jacobians of the 2D→3D map to derive the fundamental forms (key quantities in differential geometry) truly fascinating! I have big hopes for this to change the future of geometry processing.”
For Federico Tombari, he said, “a main take-away is that a lot of the research presented leverages large "foundation" models, which are now directly influencing more and more also the 3D computer vision community. In particular, the use of large pre-trained Diffusion Models and feature embeddings from pre-trained Vision-Language Models, used across the board for classic "3D" tasks such as 3D reconstruction from one or more views and 3D object detection / semantic segmentation.”
When thinking about the benefits of attending workshops hosted by ELLIS Programs like this, Federico stated,
“With conferences in the area of Computer Vision and Machine Learning growing in size, these types of workshops and events become more and more useful, as they offer a unique opportunity to interact, discuss and deep dive. The exchange and networking that arises from these events is also extremely valuable, as it offers an opportunity for PIs to initiate new collaborations, including leveraging tools offered by ELLIS (e.g. PhD co-supervision).”
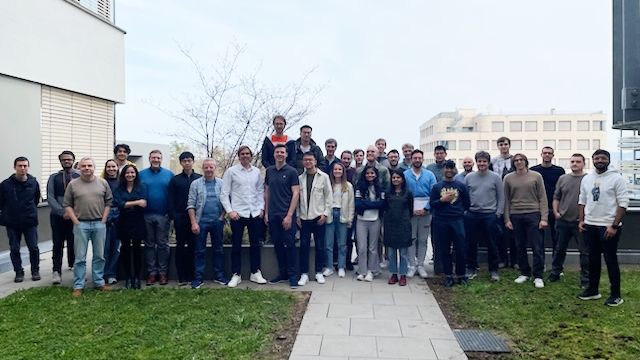