“Machine learning methods have the potential to drastically reduce the time and cost involved in molecular discovery.”
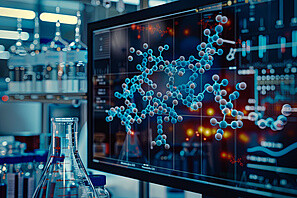
Image credit: AdobeStock_773765254 /MPI-IS/A. Posada
The discovery of new molecules with desired functions holds immense potential for addressing global challenges such as pandemics, food security and climate change. It leads to new medicines, secures the world’s food supply via agrochemicals, and enables a sustainable energy conversion. However, the discovery of new molecular materials optimized for a particular purpose often takes up to a decade and is highly cost-intensive.
The ELLIS Program ‘Machine Learning for Molecule Discovery’ aims to accelerate this process by working at the intersection of machine learning and molecular science in Europe. It focuses on establishing a dialogue between domain experts and machine learning researchers to ensure that machine learning positively impacts real world scenarios.
Three top researchers from different European countries lead the program: Francesca Grisoni, Assistant Professor in the Department of Biomedical Engineering at the Eindhoven University of Technology, Günter Klambauer, Associate Professor for Artificial Intelligence in Life Sciences at the LIT AI Lab and the Institute for Machine Learning of the Johannes Kepler University Linz, and Klaus-Robert Müller, Full Professor and Chair of the Machine Learning Department at the Technical University of Berlin.
The three program heads jointly provided the answers below explaining the transformative role of machine learning for molecular discovery, their program's objectives and plans for the future.
Could you give us an insight into your research field: How do machine learning methods accelerate molecular discovery, and what specific challenges do they help overcome in comparison to traditional methods?
Machine learning methods have the potential to drastically reduce the time and cost involved in molecular discovery. Traditional methods often rely on trial-and-error experimentation and heuristic-driven approaches, which can be both time-consuming and resource-intensive. Machine learning, on the other hand, can process vast amounts of data and identify patterns that are not immediately obvious to human researchers. This allows for the prediction of molecular properties, generating promising molecules and materials, suggesting efficient synthesis routes, and supplying new molecular representations, all of which accelerate the screening process of potential candidates.
What are examples of successful applications of machine learning methods in molecular discovery?
One notable example is the discovery of novel drug candidates. Machine learning models have been used to predict the activity of potential drugs against specific targets, leading to the identification of promising compounds that were later validated experimentally, with strongly improved hit rates. Another example is the prediction of the 3D structure of proteins by DeepMind’s AlphaFold, which allowed unprecedented insights into molecular biology. Further successes are the employment of ML-based retro-synthesis methods and language models that understand chemistry.
What are the specific objectives of your ELLIS program, and how do you envision it contributing to advancing computational molecular science?
Our primary objective is to foster collaboration between machine learning experts and molecular scientists to drive innovation in molecule discovery. We aim for a fundamental understanding and insights into molecules and their dynamics. Furthermore, we will develop new machine learning models tailored specifically for molecular applications and create benchmarks for evaluating their performance. This will ensure that the models are not only theoretically sound but also practically useful. We also aim to promote the adoption of machine learning methods in molecular science through training and education. By organizing workshops, seminars, and courses, we want to equip researchers with the skills they need to leverage these powerful tools in their own work. This will help in building a community of experts who can push the boundaries of what is possible in molecular discovery.
ELLIS programs connect researchers across Europe and foster cross-national collaboration. How does your program facilitate dialogue between European domain experts and machine learning researchers? What are ongoing projects?
We facilitate dialogue through a series of collaborative workshops and symposia where researchers from different fields can share their knowledge and ideas. Our flagship event is the yearly ELLIS Machine Learning for Molecules workshop which is organized alongside NeurIPS in December. These events are designed to be highly interactive, encouraging participants to discuss their challenges and brainstorm potential solutions together. We also promote joint research projects that bring together experts from different countries and disciplines. Ongoing projects, in which program members are involved are the European Horizon project ELISE, the MSCA projects Advanced Machine Learning for Innovative Drug Discovery (AIDD) and Explainable AI for Molecules - AiChemist. There are many more non-funded, in-official projects by program members that resulted in a series of publications.
In what ways do you foresee the program evolving in the future? Do you have any particular plans or wishes?
We foresee that the program evolves into a large network and platform that also connects pharmaceutical companies, agrochemical firms, and other relevant industries, such that we can ensure that our research has a direct impact on real-world applications. Our wish is to create a thriving community of multi-disciplinary researchers with an expertise at the intersection of machine learning and molecular sciences who are passionate about using artificial intelligence to solve some of the biggest challenges in these fields. Research at this intersection and in this interdisciplinary field is highly challenging, and therefore this program strives to foster an environment where collaboration and innovation are the norms, and where groundbreaking discoveries are made regularly. With the support of ELLIS and our partners, we are confident that we can achieve this vision.
More information
View all members of the ELLIS Program ‘Machine Learning for Molecule Discovery’ here.
ELLIS research programs focus on areas with the potential to move the needle in modern AI - from basic research in theory and algorithms to applications in health and climate sciences. They include outstanding researchers as ELLIS Fellows and Scholars. Learn more about all 16 ELLIS Programs here.
ELLIS program workshops are often supported by the EU-funded ELISE project which is building on ELLIS.
Find ELLIS contact details here.
Follow ELLIS
Follow ELLIS on X, LinkedIn, Mastodon and Facebook, and subscribe to the monthly ELLIS newsletter.