Exploring Overparametrization, Regularization, and Uncertainty in Machine Learning: Insights from the Oberwolfach Workshop
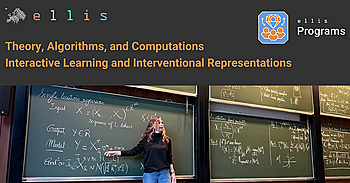
The Oberwolfach workshop, jointly organized by the ELLIS Program for Theory, Algorithms, and Computations and the Program for Interactive Learning and Interventional Representations, brought together researchers to explore key theoretical challenges in machine learning, including overparametrization, regularization, and uncertainty, fostering deep discussions and collaboration.
From January 26 to 31, 2025, the prestigious Mathematische Forschungsinstitut Oberwolfach (MFO) in Germany hosted a high-level workshop on "Overparametrization, Regularization, Identifiability, and Uncertainty in Machine Learning." Organized jointly by the ELLIS Program for Theory, Algorithms, and Computations and the Program for Interactive Learning and Interventional Representations, the event gathered 48 leading researchers from Europe, Canada, and the United States. With a particular focus on fostering collaboration among early-career scientists, the workshop provided a unique forum for deep theoretical discussions on the evolving landscape of machine learning.
A Unique Collaborative Atmosphere
Unlike large-scale conferences where discussions often remain surface-level due to time constraints, this workshop fostered an environment conducive to in-depth exchanges. Featuring 29 talks, two plenary discussions, and numerous informal sessions, the event facilitated conversations that delved into the fundamental principles shaping machine learning theory today, ranging from the historical development of learning theory to the social responsibility of theory researchers.
Researchers explored critical aspects such as the effects of overparametrization on model generalization, the role of regularization in improving stability, and challenges in ensuring model identifiability and uncertainty quantification. Shuo Huang, a Postdoctoral Fellow at the Machine Learning Genoa Center (MalGa), reflected on his experience:
I appreciated the invitation to the wonderful MFO workshop, which marked my first visit to Oberwolfach. Bringing together researchers with shared interests in the theory of machine learning fosters deep discussions that are often hard to find in large conferences. I was truly fascinated by the diverse and cutting-edge research topics presented.
Academic Talks Explored Generative AI, Algorithmic Influence, and Stochastic Modeling
Academic talks formed the main part of the program. Participants discussed wide-ranging topics. For example, ELLIS Fellow Francis Bach (INRIA) offered a take on how to generalize the recently popular generative architectures from images to more general, particularly scientific data.
ELLIS Scholar Celestine Mendler-Dünner (ELLIS Institute Tübingen) provided insights on the ability of small but well-organized groups within a population to change the decisions of algorithms in their favor by actively mislabeling themselves in the training data.
And ELLIS Scholar Gergely Neu (Universitat Pompeu Fabra) discussed the mathematical foundations of measuring similarity between stochastic processes, giving hints to novel, powerful modelling paradigms for dynamical systems.
The Value of Informal and Interdisciplinary Exchanges
One of the workshop's highlights was its emphasis on informal interactions, which proved invaluable for early-career researchers seeking to engage with the broader AI and ML theory community. Frederik Künstner, Postdoctoral Researcher at INRIA Paris, emphasized the importance of such gatherings:
As a junior researcher, this workshop provided a great opportunity to connect with the European machine learning theory community. It is rare to have such a look at the behind the scenes of research and to hear how experts in diverse sub-specialties think about broader problems in the field. In a field that moves so fast, discussions on how to identify important research directions - before they take the form of theorems - have been valuable in understanding the bigger picture. Workshops like this are unique in providing an atmosphere conductive to informal exchanges that are difficult to find in the larger and shorter formats of typical machine learning conferences.
Looking Ahead
With machine learning theory advancing at an unprecedented pace, workshops like the one at Oberwolfach are instrumental in shaping the future of the field. By creating a space for theoretical exploration, interdisciplinary collaboration, and meaningful networking, this event reinforced the value of rigorous academic exchange in navigating the complexities of modern AI.
As research into overparametrization, regularization, and uncertainty deepens, the insights and connections formed at this workshop will likely drive new breakthroughs, setting the stage for continued progress in machine learning theory.