ELLIS PhD Award
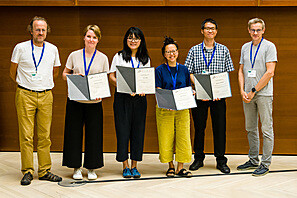
The members of the European Laboratory for Learning & Intelligent Systems (ELLIS) have announced the first six winners of the ELLIS Ph.D. Award.
The ELLIS Ph.D. Awards are sponsored by the Kübhorth Stiftung GmbH and have been established to recognize and encourage outstanding research achievements during the dissertation phase of outstanding students working in the field of artificial intelligence and machine learning including related fields such as computer vision and robotics. This year, the awards were given for the previous three years, thereafter they will be given annually.
From a set of very strong nominations, an international committee of renowned experts chose the following scholars as ELLIS Ph.D. Award winners:
- Maria Barrett (University of Copenhagen, Denmark)
- Wittawat Jitkrittum (Gatsby Unit, University College London, UK)
- Alex Kendall (Cambridge University, UK)
- Diederik Kingma (University of Amsterdam, Netherlands)
- Anastasia Pentina (Institute of Science and Technology Austria, Austria)
- Siyu Tang (Max Planck Institute for Informatics, Germany)
Maria Barrett (University of Copenhagen, Denmark)
Improving Natural Language Processing with Human Data
The dissertation makes an important theoretical contribution to the field of NLP by showing that traces of human processing, particularly eye-tracking data, can be used to improve models for a range of language processing tasks. It represents impressive innovative work, makes significant novel contributions to various NLP tasks and each of the studies presents clear hypotheses and follows a well-justified methodology. (...) Thus, the thesis as a whole advances the state- of-the-art NLP research by suggesting what can be defined a new and promising modelling paradigm.
Wittawat Jitkrittum (Gatsby Unit, University College London, UK)
Kernel-Based Distribution Features for Statistical Tests and Bayesian Inference
Wittawat Jitkrittum's thesis addresses the problem of finding computationally efficient and informative features for representing and comparing distributions, while making as few assumptions as possible. The proposed two-sample test, independence test, and goodness-of-fit test are the first to combine consistency against all alternatives, adaptivity to maximise test power, efficiency (linear runtime), and informativeness (showing why a decision is made).
Alex Kendall (University of Cambridge, UK)
Geometry and Uncertainty in Deep Learning for Computer Vision
Alex Kendall’s dissertation introduced a number of exceptional new research contributions in the field of computer vision and deep learning. In particular, it advanced state-of-the-art in semantic segmentation, localisation, stereo vision and scene understanding using ideas from multi-view geometry and probabilistic modelling.
Diederik Kingma (University of Amsterdam, Netherlands)
Variational Inference and Deep Learning: A New Synthesis
Diederik proposed novel efficient solutions to the problems in variational (Bayesian) inference, generative modeling, representation learning, semi-supervised learning, and stochastic optimization. Among the proposed methods are the Adam optimization method, the Variational Autoencoder using the Reparameterization Trick, Inverse Autoregressive Flows, Weight Normalization and the Local Reparameterization Trick.
Anastasia Pentina (Institute of Science and Technology Austria, Austria)
Theoretical foundations of multi-task and lifelong learning
Anastasia Pentina contributed to expanding the understanding of potential benefits of information transfer in the multi-task and lifelong learning scenarios. She formally identified and analysed several scenarios of transfer learning. From the theoretical results she derived new principled multi-task and lifelong learning algorithms and illustrated their performance on various synthetic and real-world datasets.
Siyu Tang (Max Planck Institute for Informatics, Germany)
People detection and tracking in crowded scenes
Siyu Tang made significant contributions in the area of multi-person tracking and detection. She proposed novel and rigorous mathematical formulations based on the Minimum Cost Multicut models that perform well for real-word and challenging visual scenes and thus have defined the state-of-the-art in this area.