ELIAS: Atmospheric Machine Learning Emulation Challenge (AMLEC)
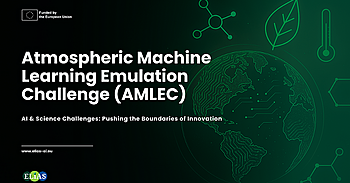
The Atmospheric Machine Learning Emulation Challenge (AMLEC) invites researchers, data scientists, and practitioners in the fields of remote sensing, climate science, and artificial intelligence to contribute solutions to a key computational challenge in atmospheric modelling.
Organised by Jorge Vicent Servera, Gustau Camps Valls, Cesar Aybar, and Julio Contreras from the Image and Signal Processing Group (ISP) at the University of Valencia, AMLEC focuses on advancing surrogate modelling and physics-informed AI in the context of Radiative Transfer Models (RTMs).
About the Challenge
Radiative Transfer Models are fundamental tools in Earth observation and climate research. However, their high computational demands often limit their application in real-time or large-scale scenarios — especially as the volume and complexity of hyperspectral satellite data continue to increase.
Common alternatives, such as look-up tables (LUTs), can reduce computational load but are memory-intensive and lack flexibility. Machine learning-based emulators offer a promising alternative by producing fast and accurate approximations of RTM outputs.
AMLEC aims to support the development of statistical models that emulate RTM behaviour, enabling faster atmospheric data processing, improved climate simulations, and enhanced remote sensing workflows. The challenge also addresses the difficulties posed by high-dimensional inputs and the complex physics embedded in RTMs.
Key Dates
- Challenge opens: 21 April 2025
- Submission deadline: 30 June 2025