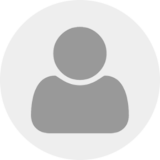
Discovering causal mechanisms in Single-Cell Omics
Alejandro Tejada (Ph.D. Student)
Single-Cell genomics allow to get a fine resolution of the internal state of a cell. Nevertheless, the dynamics and mechanisms that rule the behaviour of cells are mainly unknown. Due to the enormous number of degrees of freedom, classical scientific approaches may fail at modelling and capturing the complexity of Single-Cell biology. On contrast, Machine Learning techniques are suitable for settings in which a set of rules and relationships must be inferred from a vast amount of data. The application of Causal Machine Learning approaches to Single-Cell genomics can generate new scientific knowledge identifying biologically meaningful causal variables previously unknown and disentangle cellular mechanisms. During my Phd I will explore Single-Cell genomics from a causal point of view, trying to identify the cellular mechanisms the define the response to perturbations, cell fate, or spatial organization. My goal is to decipher the causal mechanisms that rule Single-Cell biology to expand scientific knowledge and model the response of a cell under external perturbations.
Primary Advisor: | Fabian Theis (Helmholtz Center Munich) |
Industry Advisor: | Taco Cohen (Qualcomm AI Research) |
PhD Duration: | 01 July 2022 - 01 July 2025 |