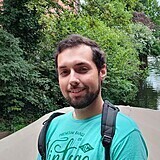
Bayesian networks for interpretable machine learning
Enrique Valero (Ph.D. Student)
The project orbit arounds the state-of-the-art topic of explainable artificial intelligence, that aims toward making intelligent system easier to understand. Most of the current literature covers topics related to neural networks and deep learning applied to supervised learning tasks. However, simpler models with good performance are usually ignored, even though explainability is still desirable and other type of tasks are underrepresented in the literature. As such, my research will focus on using Bayesian for improved machine learning explainability and how to increase the interpretability of these models themselves. Bayesian networks can provide a strong statistical and mathematical component to a field that is currently highly based in heuristics and approximantions and, furthermore, these models can perform a wide variety of tasks, such as supervised and unsupervised learning, anomaly detection and time series analysis.
Primary Host: | Pedro Larrañaga (Universidad Politécnica de Madrid) |
Exchange Host: | Bernd Bischl (LMU Munich) |
PhD Duration: | 01 October 2022 - 01 August 2025 |
Exchange Duration: | 01 September 2024 - 01 April 2025 - Ongoing |